Research Focus
Spatio-Temporal Data Mining, Urban Computing, Smart City
Urban computing is a process of acquisition, integration, and analysis of big and heterogeneous data generated by a diversity of sources in urban spaces, such as sensors, devices, vehicles, buildings, and human, to tackle the major issues that cities face, e.g. air pollution, increased energy consumption and traffic congestion. Urban computing connects unobtrusive and ubiquitous sensing technologies, advanced data management and analytics models, and novel visualization methods, to create win-win-win solutions that improve urban environment, human life quality, and city operation systems. Urban computing also helps us understand the nature of urban phenomena and even predict the future of cities.
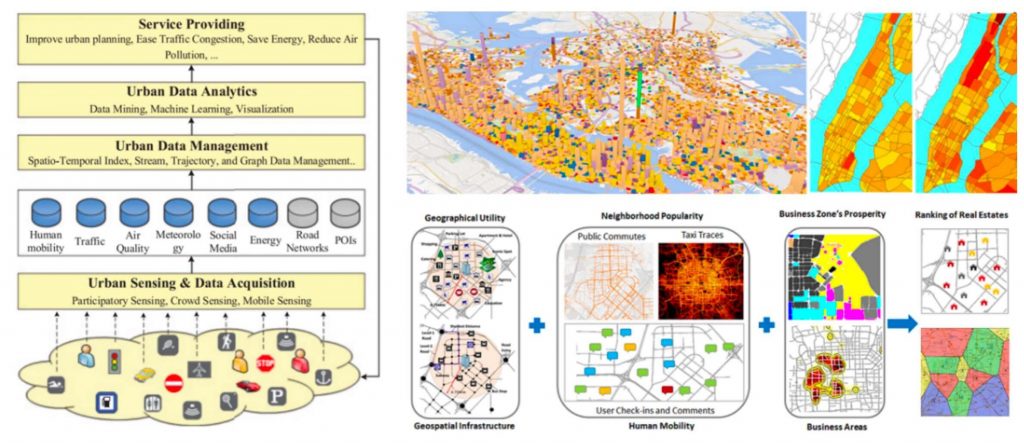
Spatio-Temporal Artificial Intelligence, ST Representation Learning
With rapid advances in new-generation information technologies such as the IoT, 5G, and mobile Internet, Spatio-Temporal (ST) data are growing explosively in the physical world. In contrast to image, text, and voice data, ST data often present unique spatio-temporal characteristics, such as spatial distance and hierarchy, as well as temporal closeness, periodicity, and trend. Spatio-Temporal AI is a proprietary AI technology for learning the representations of ST data, where AI meets conventional city-related fields, like transportation, civil engineering, environment, and economy, in the context of space-time. The applications of spatio-temporal AI can be categorized into four categories, including the modeling of ST trajectories, ST grid data, ST graphs, and ST series. Our group has been exploring the methodologies of the above four categories in various applications.
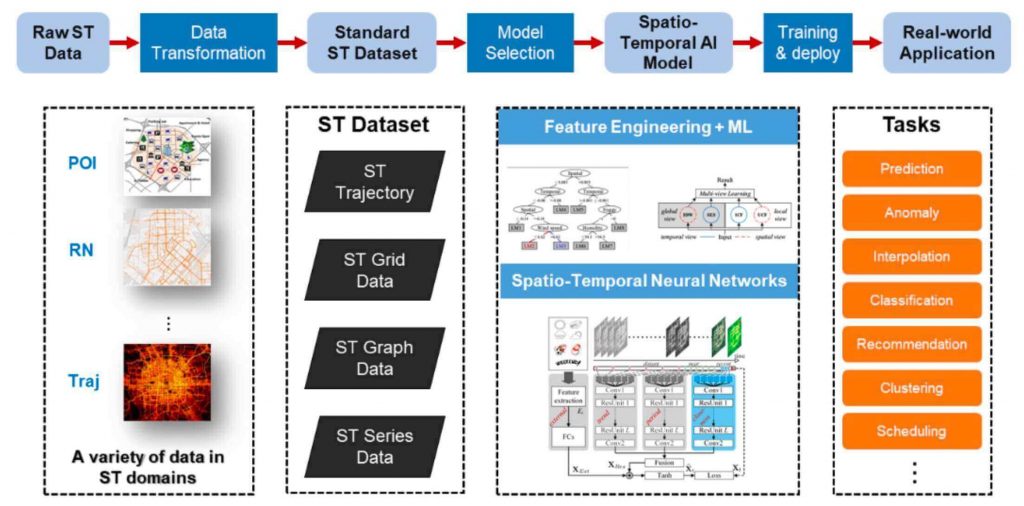
Time Series Analysis
Time series data are becoming ubiquitous in numerous real-world applications, e.g., IoT devices, healthcare, wearable devices, smart vehicles, financial markets, etc. Given the availability of massive amounts of data, their complex underlying structures/distributions, together with the high-performance computing platforms, there is a great demand for developing new theories and algorithms to tackle some fundamental challenges (e.g., representation, classification, prediction, causal analysis, etc.) in time series. Time Series Analysis involves the application of AI or statistical algorithms to analyze patterns (e.g., seasonality, trend) within the data and make predictions about future values.
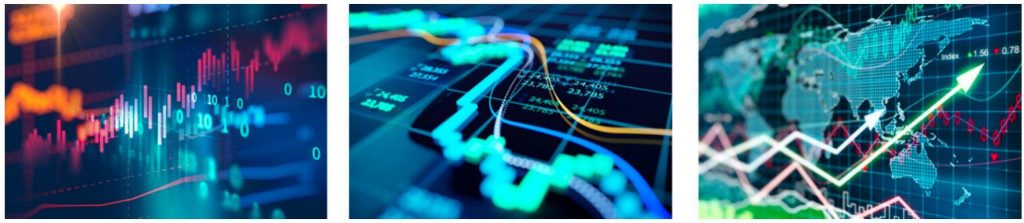
AI for Science, especially in the fields of environment and earth science
AI for Science refers to the use of AI techniques in scientific research and discovery. It involves the application of machine learning, natural language processing, computer vision, and other AI technologies to analyze large amounts of data and generate insights that can accelerate scientific progress. AI for Science has the potential to transform the way scientists conduct research, from drug discovery to climate modeling, by enabling faster and more accurate data analysis, hypothesis testing, and prediction of future outcomes.
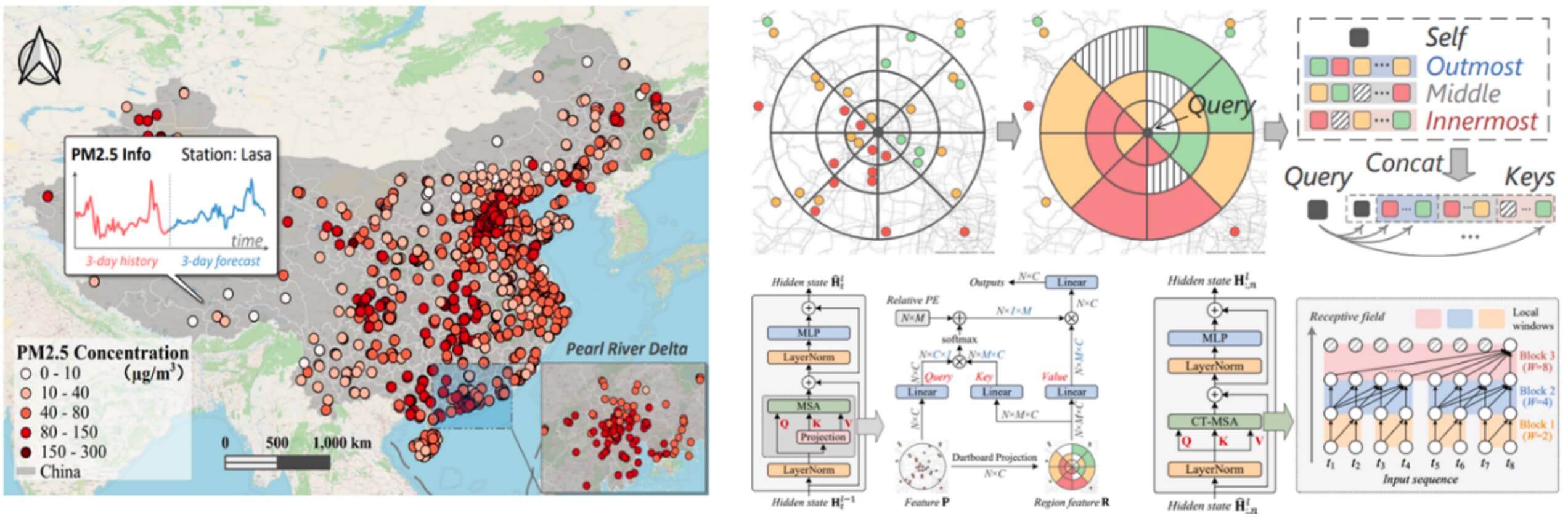